Harnessing the Power of Agriculture Dataset for Machine Learning: Unlocking Innovation in Agriculture
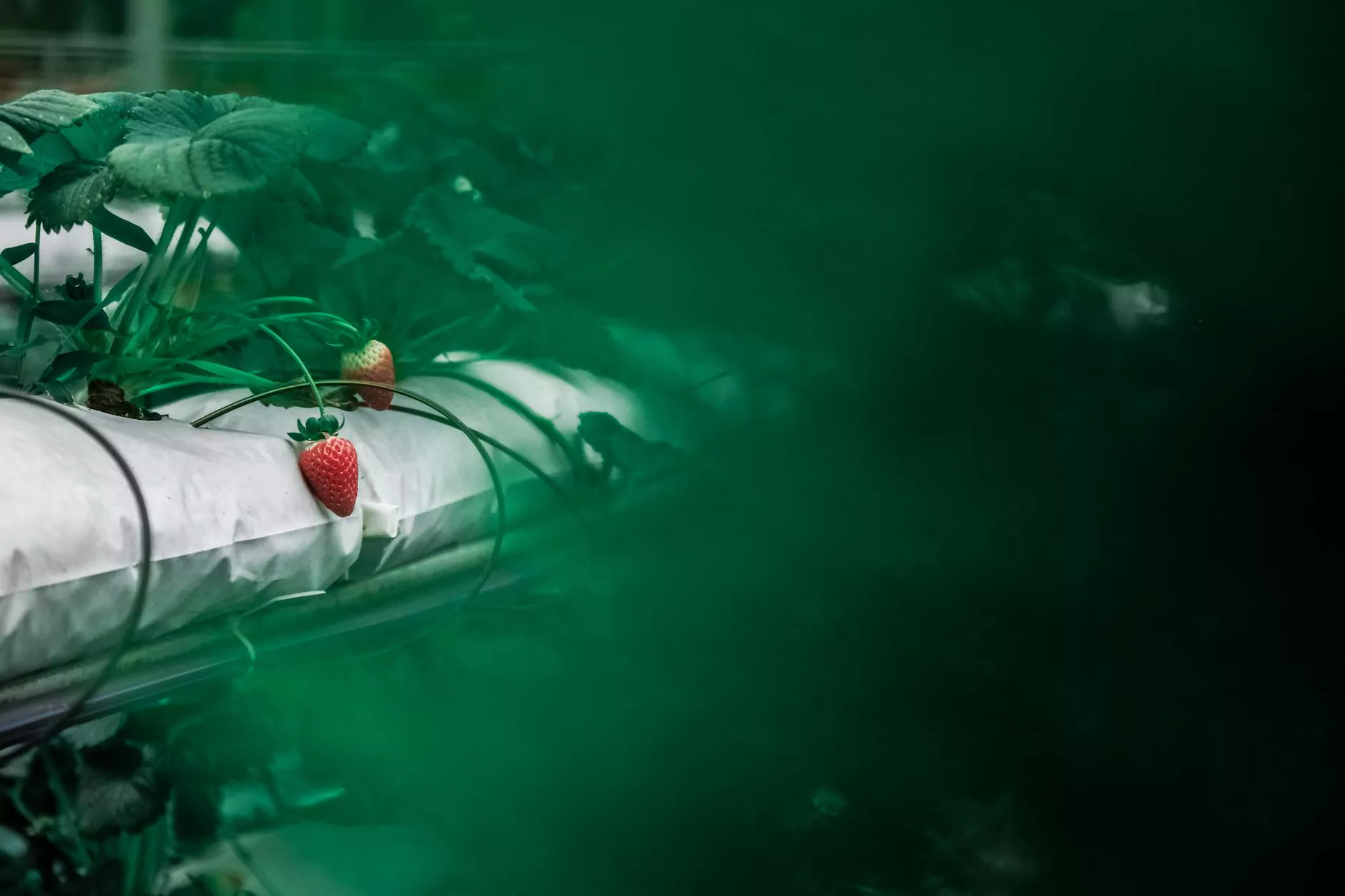
In today’s rapidly evolving technological landscape, the integration of machine learning into agriculture has transformed traditional farming practices, leading to increased productivity, sustainability, and resource optimization. Central to this revolution is the availability and utilization of comprehensive agriculture dataset for machine learning. These datasets serve as the backbone for developing sophisticated algorithms that enable predictive analytics, precision farming, pest and disease detection, and resource management.
Understanding the Significance of Agriculture Datasets in Machine Learning
The success of machine learning models in agriculture hinges on the quality, diversity, and volume of data available. An agriculture dataset for machine learning typically contains a rich collection of biological, environmental, and operational data points, such as soil composition, crop health images, weather conditions, satellite imagery, and sensor readings.
Why are these datasets crucial? Because they allow models to identify complex patterns, forecast yields, detect anomalies, and optimize decision-making. Without robust datasets, even the most advanced algorithms cannot achieve high accuracy or reliable performance, which underscores the importance of investing in high-quality data collection and management.
Types of Agriculture Data Used in Machine Learning Projects
- Sensor Data: Soil moisture, temperature, pH levels, and nutrient content collected via IoT devices.
- Imagery Data: Satellite images, drone photography, and multispectral images used for monitoring crop health and pest infestations.
- Weather Data: Real-time weather metrics such as rainfall, humidity, wind speed, and temperature.
- Crop and Plant Data: Growth patterns, phenological stages, and yield records.
- Operational Data: Machinery usage logs, irrigation schedules, and fertilization records.
- Biological Data: Pest populations, disease prevalence, and genetic information of crops and livestock.
Key Features of High-Quality Agriculture Datasets for Machine Learning
Developing effective machine learning models requires datasets that possess certain essential qualities:
- Completeness: Data should cover all relevant aspects of the agricultural environment to provide a comprehensive view.
- Accuracy: Precise measurements and annotations are vital for training reliable models.
- Consistency: Data collection methods should be standardized to reduce variability and bias.
- Timeliness: Up-to-date data enables models to adapt to current conditions and trends.
- Diversity: Including different crop types, climates, and regions enhances the generalizability of models.
The Role of Data Providers in Developing Agriculture Datasets
Leading companies like keymakr.com specialize in creating tailored datasets for various applications, including agriculture dataset for machine learning. These organizations utilize advanced data acquisition techniques, such as drone mapping, remote sensing, and IoT sensor deployment, to compile extensive datasets that meet rigorous quality standards.
By collaborating with agricultural experts, data scientists, and technology providers, data providers ensure datasets are annotated accurately, structured suitably for machine learning workflows, and aligned with specific project objectives.
Applications of Agriculture Datasets in Machine Learning
Harnessing large-scale, high-quality datasets empowers multiple innovative applications in agriculture:
1. Precision Farming
Using geospatial and sensor data, machine learning models can recommend optimal planting times, irrigation schedules, and fertilization strategies, improving crop yields while reducing input costs.
2. Disease and Pest Detection
Image datasets of plants affected by pests or diseases allow models to automatically identify issues early, enabling targeted interventions and minimizing crop losses.
3. Crop Yield Prediction
By analyzing weather patterns, soil conditions, and historical yield data, models can accurately forecast harvest outcomes, aiding farmers in planning and resource allocation.
4. Weather Forecasting and Climate Modeling
Integrating weather data into machine learning models enhances climate resilience strategies and helps in adapting farming practices to changing conditions.
5. Soil Management
Datasets related to soil health assist in developing recommendations for crop rotation, soil amendments, and conservation practices.
Leveraging Keymakr.com for Superior Agriculture Datasets
Keymakr specializes in delivering customized datasets that cater specifically to the needs of agricultural machine learning projects. Their expertise spans data collection, annotation, and quality assurance, ensuring that clients receive datasets that are not only comprehensive but also optimized for high-performance model training.
Whether you require satellite imagery for large-scale analysis or sensor data for precision irrigation, keymakr's capabilities cover a wide spectrum of data needs. Their commitment to data integrity and technological innovation makes them a trusted partner for organizations aiming to push the boundaries of agricultural intelligence.
Challenges in Developing and Using Agriculture Dataset for Machine Learning
Despite the numerous advantages, creating and deploying effective datasets in agriculture involves challenges such as:
- Data Quality and Noise: Environmental variability and sensor inaccuracies can introduce noise, affecting model performance.
- Data Scarcity: Some regions or crops may lack sufficient data, hindering model training.
- Data Privacy and Ownership: Farmers and organizations may have concerns over data sharing and intellectual property rights.
- Integration Complexity: Combining heterogeneous data sources requires advanced data integration techniques.
- Cost of Data Collection: High-quality data acquisition still entails significant investments in infrastructure and technology.
Overcoming these hurdles involves adopting standardized data collection protocols, investing in robust data infrastructure, and fostering collaboration among stakeholders in the agricultural ecosystem.
Future Trends in Agriculture Dataset Development for Machine Learning
The future of agriculture dataset for machine learning is poised to be shaped by innovations like:
- Automated Data Collection: Use of drones, autonomous vehicles, and IoT devices for real-time, continuous data gathering.
- Advanced Data Annotation Tools: AI-assisted labeling to accelerate dataset preparation.
- Data Sharing Platforms: Collaborative cloud-based repositories to foster data democratization.
- Integration of Multi-Modal Data: Combining imagery, sensor data, and genetic information for holistic models.
- Focus on Sustainability: Datasets aimed at promoting environmentally friendly practices and climate adaptation.
Conclusion: Empowering Agriculture through Data-Driven Innovation
The agriculture dataset for machine learning is undeniably a catalyst for the transformation of modern agriculture. Its ability to provide insights, optimize operations, and foster sustainable practices is invaluable for farmers, researchers, and industry stakeholders alike. As technological advancements continue to accelerate, the demand for high-quality datasets will only increase, making it essential for organizations to invest in data infrastructure and partnerships that facilitate data excellence.
Leading data providers like keymakr.com are at the forefront of this movement, delivering tailored, premium datasets that enable innovative, data-driven farming solutions. Embracing these resources will empower the future of agriculture—smarter, more sustainable, and resilient than ever before.