Unlocking the Power of Image Datasets for Object Detection
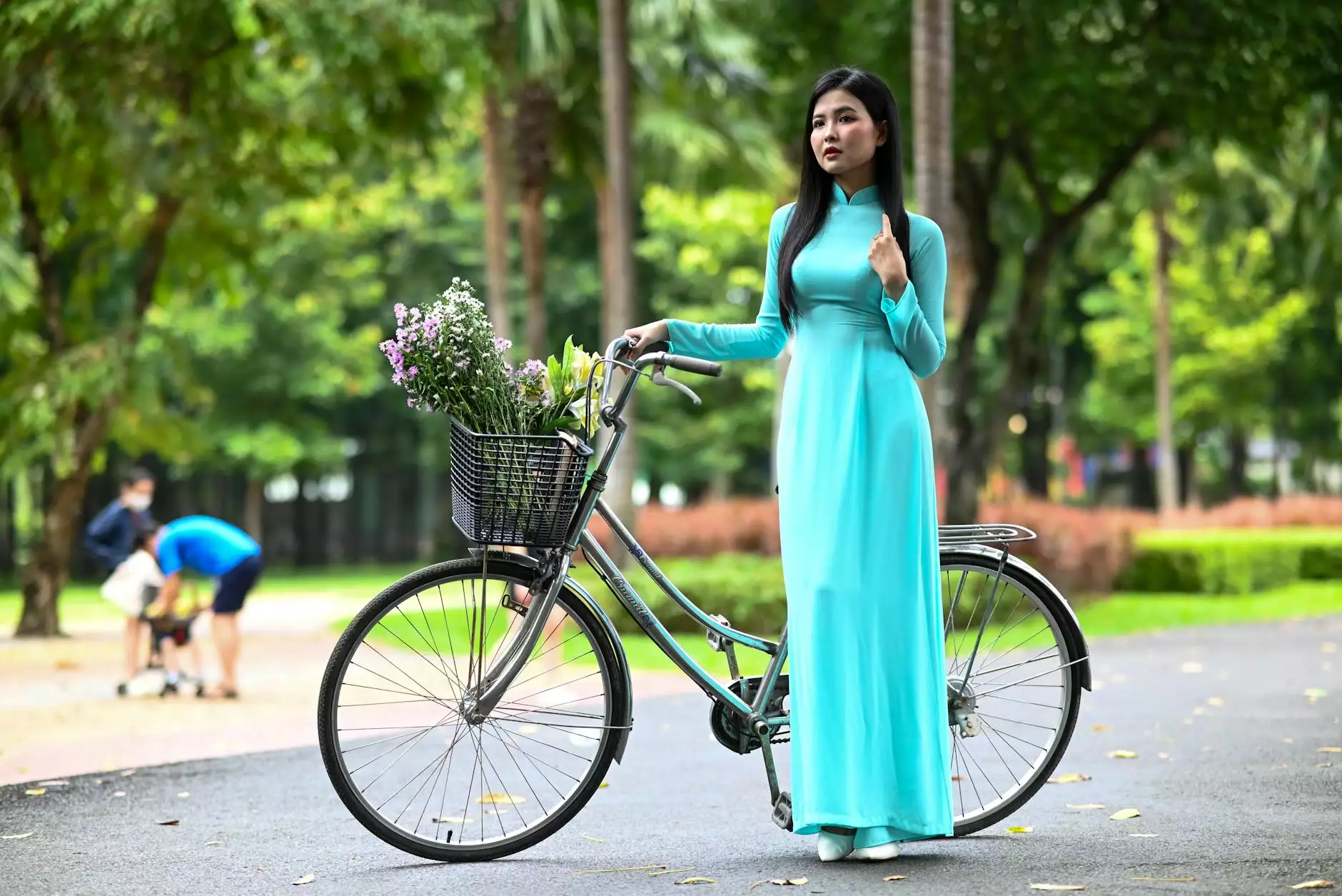
Understanding Image Datasets
In the realm of artificial intelligence and machine learning, the quality of data is paramount. Among the many types of data, image datasets play a crucial role, especially in object detection applications. Businesses are increasingly realizing the value of these datasets in enhancing their computer vision models.
What is Object Detection?
Object detection is a computer vision task that involves identifying and locating objects within an image. It goes beyond simple image classification by not only determining what objects are present, but also specifying their locations. This ability is vital across various industries including:
- Automotive - for self-driving car systems
- Healthcare - for tumor detection in medical imaging
- Retail - for inventory management through smart cameras
- Security - for surveillance systems that recognize potential threats
The Importance of Quality Image Datasets
The efficacy of object detection models heavily relies on the quality and diversity of the training datasets used. High-quality image datasets lead to better generalization, meaning the model can accurately identify objects in new, unseen images. Here are some key factors that contribute to a quality dataset:
- Diversity of Scenes - The dataset should cover a wide range of environments.
- Variety of Object Classes - Include a diverse set of objects for comprehensive learning.
- Annotation Accuracy - Precise and accurate labels are critical for training.
- Image Quality - High-resolution images help in better feature extraction.
Data Annotation: The Backbone of Image Datasets
One of the vital processes in creating image datasets for object detection is data annotation. Data annotation involves adding metadata to images to train machine learning models effectively. Keylabs.ai offers advanced data annotation tools and platforms designed to streamline this process.
There are several annotation techniques used depending on the requirements:
- Bounding Box Annotation - Drawing boxes around target objects.
- Polygon Annotation - Creating more precise shapes around complex objects.
- Semantic Segmentation - Classifying every pixel in an image for detailed recognition.
- Instance Segmentation - Identifying and segmenting each instance of an object in images.
Leveraging Keylabs.ai for Superior Image Datasets
At Keylabs.ai, we specialize in providing data annotation solutions that empower businesses to build accurate and reliable object detection systems. Here’s how our platform stands out:
1. Scalable Solutions
We understand that businesses grow, and so do their data annotation needs. Our platform scales seamlessly to handle small to large projects without compromising quality.
2. Expertise in Various Domains
Our team consists of experts in different industries, ensuring that the annotations are contextually relevant and accurate. This domain-specific expertise is crucial for creating robust image datasets for object detection.
3. Advanced Tools and Technology
We utilize state-of-the-art tools that enhance the annotation process, making it efficient and fast. This leads to quicker turnaround times, allowing businesses to bring their products to market sooner.
4. Quality Assurance
Quality is non-negotiable at Keylabs.ai. We implement strict quality control measures to ensure that every dataset is annotated to the highest standards. Our double-check mechanism significantly reduces errors, ensuring your image dataset for object detection is top-notch.
Best Practices for Creating Image Datasets
When creating image datasets for object detection, it’s important to follow best practices to ensure that your dataset is effective for training machine learning models. Consider the following:
- Define Your Objectives Clearly - Know what you want to achieve with the dataset.
- Collect Diverse Images - Gather images from varied sources to enhance model learning.
- Ensure Quality Annotation - Use skilled annotators or reliable tools to maintain quality.
- Regularly Update the Dataset - Keep the dataset fresh with new images to improve model performance over time.
Case Studies: Successful Implementations
Numerous organizations have transformed their operations through the effective use of image datasets for object detection. Let’s explore a few successful applications:
Healthcare Sector
A leading healthcare provider implemented an object detection system for early tumor detection using annotated image datasets. By employing our data annotation platform, they achieved a 15% increase in diagnostic accuracy in just six months.
Retail Industry
A major retail chain utilized object detection to manage inventory in its stores. By employing image datasets, they reduced inventory discrepancies by 20%, leading to significant cost savings.
The Future of Object Detection with Image Datasets
The future of object detection is promising, powered by advancements in artificial intelligence and machine learning. The growing availability of large-scale image datasets will continue to enhance model capabilities, especially in:
- Real-time Processing - Faster object detection with improved computational algorithms.
- Increased Accuracy - Enhanced learning with more diverse datasets.
- Integration of 3D Data - Incorporating depth perception for better object detection.
Conclusion
In conclusion, the power of image datasets for object detection cannot be overstated. By investing in high-quality data annotation through platforms like Keylabs.ai, businesses can harness the full potential of computer vision technology. Whether in healthcare, retail, or security, the right image dataset can lead to significant improvements in operational efficiency and decision-making capabilities. Embrace the future of object detection today!
For more information and to enhance your object detection projects, visit Keylabs.ai